
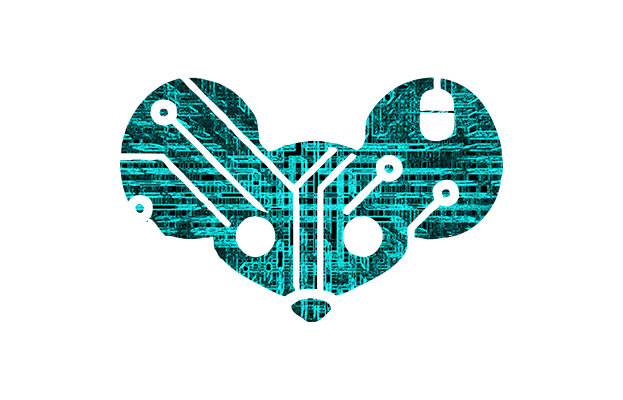
Here is the more burning question: What is worse? Case “It was not made to design standards”: Then boing might have a problem in their manufacturing processes, which is going to have ramifications on the entire fleet. This would be bad, but fixable.
Case “It was made to design standards”: In that case you only have a problem with this one type of jet, but you have a problem in your fundamental design, which might ground the entire fleet (again).
exactly: It’s “open source” like android. The core android is open source (in many cases because they are required to), but that does not include anything that makes the actual system work for normal users. The core android is open source (“Android Open Source Project”), but that includes practically nothing: Essentially the stuff that is in there are things that have to be open source (like the linux kernel they use). However, if you want to have the system “practically useable” you need a lot more, which is usually the “Google Mobile Services”, which are proprietary. You are also generally required to install all items in the GMS, i.e. even if you only need the play store, you still have to install google chrome.
Further, the android name and logo are trademarked by google, so even if you want to roll your own android, you would not be allowed to call it android. WearOS is essentially the same thing: The android subsystem is open, the actual thing you call WearOS (plus trademarks, etc.) are not.